Unlocking Business Potential: The Role of Image Annotation Tools in Machine Learning
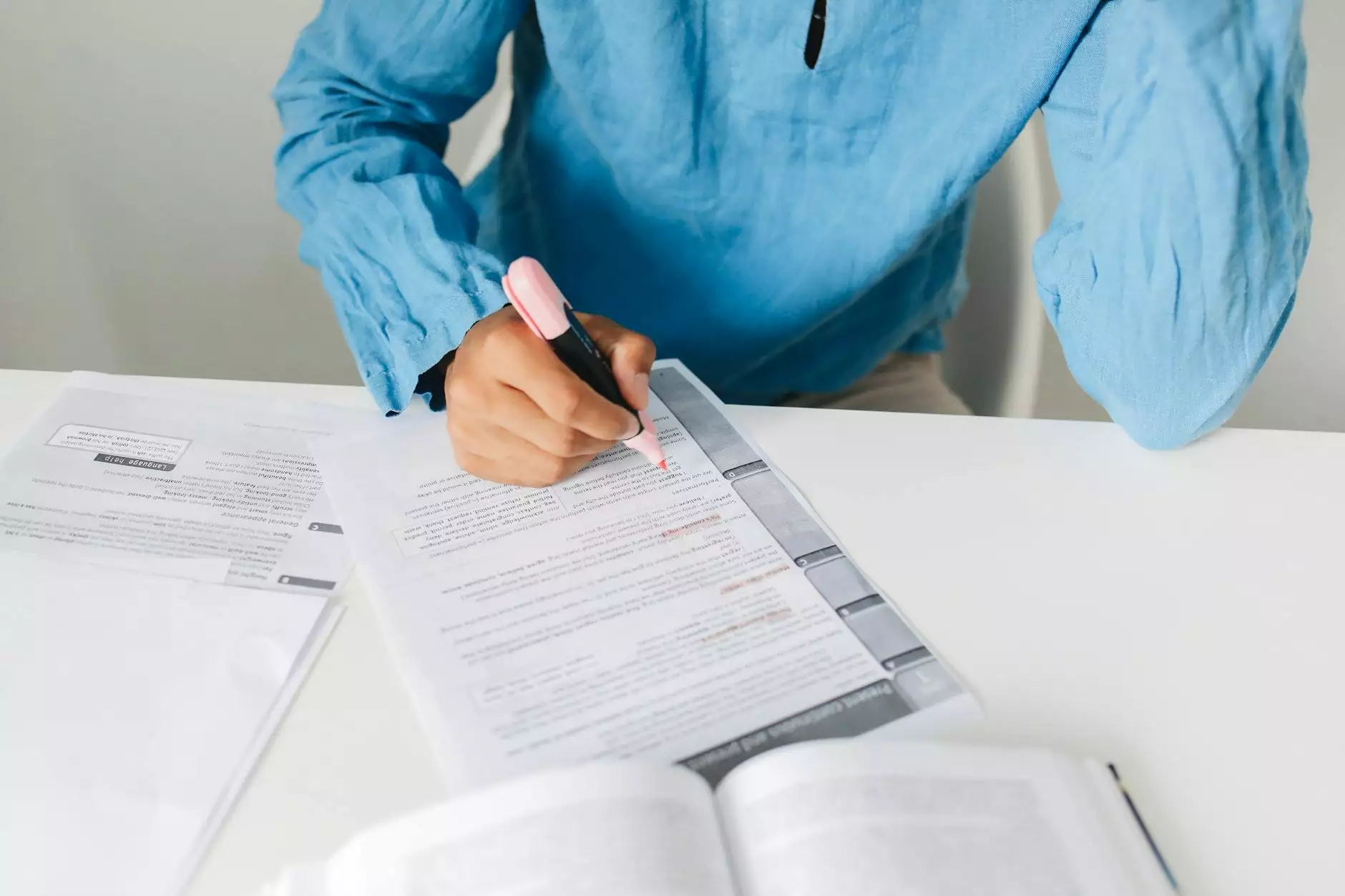
The rapid evolution of technology has heralded significant advancements in many fields, particularly in machine learning and artificial intelligence (AI). Within these domains, one vital component is often overlooked: image annotation tools. As businesses strive to harness the power of machine learning, understanding the importance of these tools becomes paramount to driving innovation and efficiency.
Understanding Image Annotation in Machine Learning
At its core, image annotation refers to the process of labeling images to create datasets that machine learning models can utilize. This labeling transforms unstructured data into structured data that algorithms can analyze and learn from. Image annotation is particularly crucial for tasks that involve computer vision, where machines are trained to recognize and interpret visual inputs.
The Impact of Image Annotation in Business
The significance of image annotation tools can be illustrated by examining their applications across various sectors:
- Healthcare: Medical imaging relies heavily on accurate annotations for diagnostic purposes. Annotations facilitate the training of AI models to detect anomalies in X-rays, MRIs, and other imaging techniques.
- Retail: E-commerce businesses utilize image annotation to improve product placement and visual search capabilities. Annotations enable robust search functionalities, enhancing the customer experience.
- Automotive: In the realm of autonomous vehicles, image annotation is crucial for understanding road conditions, recognizing pedestrians, and interpreting traffic signals.
- Agriculture: Drones equipped with AI-driven image annotation can assess crop health and yield, assisting farmers in making informed decisions.
Why Businesses Should Invest in Image Annotation Tools
For businesses looking to leverage machine learning, investing in the right image annotation tool is essential. Here’s why:
1. Enhanced Data Quality
The quality of your machine learning models is heavily dependent on the quality of the data used for training. Good image annotation ensures crisp and accurate labeling of images, which leads to better model performance. High-quality annotated datasets are the backbone of successful machine learning projects.
2. Increased Efficiency
Manually annotating images can be a time-consuming and tedious process. However, utilizing effective image annotation tools, such as those from keymakr.com, can streamline this process significantly. Automated annotation solutions can reduce the time taken to label datasets, allowing businesses to focus on core activities.
3. Scalability
Growing businesses often struggle with scaling their operations. Image annotation tools offer the ability to annotate thousands of images quickly, enabling businesses to expand their machine learning projects without being bottlenecked by the data labeling stage.
4. Competitive Advantage
In today’s competitive landscape, being the first to leverage advanced technologies can set a business apart. By utilizing image annotation tools early on, companies can develop superior machine learning models that provide better insights, thus gaining a strategic edge.
Key Features to Look for in Image Annotation Tools
When selecting an image annotation tool for machine learning, consider the following features:
- User-Friendly Interface: The tool should be intuitive, allowing team members to onboard easily and start working efficiently.
- Support for Multiple Annotation Types: Different projects may require various annotation types (bounding boxes, segmentation, landmarks, etc.), so versatility is key.
- Collaboration Capabilities: Tools that allow multiple users to collaborate in real-time enhance productivity, especially in larger teams.
- Integration with Existing Systems: Ensure that the tool can seamlessly integrate with other software solutions your business uses, facilitating a smooth workflow.
The Future of Image Annotation Tools and Machine Learning
As machine learning continues to evolve, the role of image annotation tools is poised to grow even more significant. Emerging technologies, such as AI-driven annotation, promise to make this process even more efficient. For instance, tools that utilize deep learning can learn from a dataset and automate the annotation process with minimal human intervention, drastically reducing the time and cost associated with data labeling tasks.
Ethical Considerations in Image Annotation
While leveraging image annotation tools, businesses must also be proactive about ethical considerations:
- Bias Minimization: Ensure that the dataset used for annotation is diverse and represents various demographic groups to prevent biased outputs from machine learning models.
- Privacy Protection: Annotations involving sensitive images (like those in healthcare) should adhere to strict privacy regulations to protect patient confidentiality.
- Transparency: Be clear about how annotated data will be used to maintain trust with stakeholders.
Case Studies: Real-World Applications of Image Annotation Tools
Several prominent companies have effectively harnessed the power of image annotation in their machine learning projects:
Healthcare Example: Zebra Medical Vision
Zebra Medical Vision uses image annotation tools to label thousands of medical images, training their AI to identify cardiovascular disease and other health issues. Their models have significantly improved diagnostic accuracy, demonstrating the transformative impact of effective image annotation in healthcare.
Retail Example: eBay
eBay has implemented image annotation to enhance its search functionality. By annotating product images, eBay allows users to perform visual searches, dramatically improving the user experience and increasing sales.
Automotive Example: Tesla
Tesla’s self-driving car technology relies on sophisticated image annotation techniques to train its vehicles’ AI systems. By continuously labeling images captured during test drives, Tesla enhances its cars' ability to navigate complex environments autonomously.
Getting Started with Image Annotation Tools
To integrate image annotation tools effectively into your business’s machine learning strategy, follow these steps:
- Identify the Use Case: Determine what you need machine learning for and how annotated images will support that need.
- Choose the Right Tool: Research and select an annotation tool that fits your team’s needs and budget.
- Train Your Team: Ensure your team knows how to use the chosen tool effectively through training and integration sessions.
- Monitor Progress: Track the performance of the annotated datasets and the subsequent machine learning models to assess effectiveness.
Conclusion
In conclusion, image annotation tools are indispensable for businesses aiming to leverage machine learning to its fullest potential. From improving data quality to enhancing operational efficiency, these tools provide the structure needed for successful AI initiatives. As we move towards a more data-driven future, incorporating image annotation as a foundational component will enable businesses to thrive in an increasingly competitive landscape. Investing in quality image annotation will not only meet the current demands but also prepare your business for the advanced AI capabilities of tomorrow.
image annotation tool machine learning